In the rapidly expanding realm of Health IoT, data analytics is the key to unlocking the full potential of connected medical devices. It transforms raw data into actionable insights, helping healthcare providers optimize patient care and improve outcomes. With the growing integration of IoT in healthcare, data analytics has the power to drive more personalized, preventive, and efficient care delivery.
Table of Contents
IoT Analytics in Healthcare: Empowering Integrated Care, Proactive Care
Through IoT analytics, healthcare providers can remotely monitor patient health via connected devices like wearable sensors and smartphone apps. This real-time data allows for a more proactive approach to treatment, as physicians can assess individual risk profiles and intervene early. However, gaps remain—especially in areas like mental health diagnosis, where data-based insights still lag behind emerging health trends.
Furthermore, challenges such as the high costs of VR-based medical education and the complexity of analyzing data from connected medical devices are slowing innovation in patient treatment. But by harnessing historical data housed in hospitals’ data centers, healthcare organizations can extract valuable insights, improving the efficacy of IoT platforms.
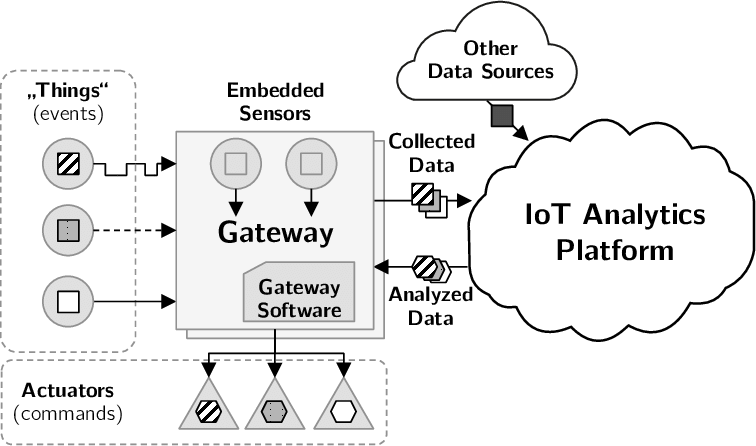
Reference: Müller, Stephan & Wiener, Patrick & Bürger, Adrian & Nimis, Jens. (2017). IoT for All: Architectural Design of an Extensible and Lightweight IoT Analytics Platform.
Market assessments
Despite global disruptions like the chip shortage and inflation, the IoT market remains poised for significant growth. As connectivity between devices expands—projected to reach 22.5 billion connections by 2022—the healthcare sector stands to benefit from exponential advances in IoT solutions and services.
However, providers must remain agile in addressing market challenges like rising costs and geopolitical uncertainties. By focusing on efficiency in data collection and IoT analytics, healthcare providers can maintain a competitive edge, driving more integrated and personalized care for patients.
The Four Pillars of IoT Analytics
IoT analytics encompasses various techniques that data scientists employ to tackle different challenges and gain valuable insights. There are primarily four key fields in IoT analytics: descriptive analytics, diagnostic analytics, predictive analytics, and prescriptive analytics.
1. Predictive Analytics:
Predictive analysis leverages the power of machine learning to estimate the probability of future events and predict their outcomes. By utilizing extensive datasets, machine learning models can anticipate upcoming events. This predictive capability enables organizations to proactively improve expected outcomes.
2. Diagnostic Analytics:
Diagnostic analysis uncovers the underlying causes and mechanisms behind various occurrences. It is particularly useful in identifying anomalies, inefficiencies, and emerging trends. For instance, when IoT devices experience performance issues, diagnostic analytics can analyze the data collected from these devices to pinpoint the root causes of failures.
3. Descriptive Analytics:
Descriptive analysis focuses on understanding real-time data obtained from connected devices and networks. It involves measuring and assessing device performance to ensure proper operation. Additionally, descriptive analytics aids in identifying anomalies and gauging internal device usage.
4. Prescriptive Analytics:
Prescriptive analytics complements predictive analysis by providing insights on influencing future outcomes. This analytical approach offers recommendations and actionable guidance based on the predictions derived from predictive analytics. It aims to optimize decision-making processes and helps organizations reduce costs.
By leveraging the power of IoT analytics across these different fields, organizations can unlock valuable insights and optimize their operations for greater efficiency and success.
Challenges and Opportunities
While Health IoT offers immense opportunities, challenges remain in managing the vast amounts of data generated by devices. The heterogeneous nature of this data, combined with storage and analysis limitations, complicates the task of extracting actionable insights. However, platforms like AWS IoT and Azure Stream Analytics are paving the way for predictive maintenance and real-time data analytics in healthcare, offering new solutions for overcoming these challenges.
In a study conducted by Amaral et al. (2016), it was found that for the adoption of 5G technology to become widespread, there is a need for a massive system capacity and powerful edge nodes. These edge nodes should be able to offload the traffic from the core network. For healthcare providers, these advancements represent an opportunity to enhance operational efficiency, improve patient outcomes, and integrate IoT data into personalized care pathways. The hospitality industry can also benefit, as IoT analytics could be applied to deliver health and wellness services in new, innovative ways—such as personalized wellness programs for guests based on real-time health data.
The Future of IoT Analytics in Healthcare
Comprehending data analytics within the realm of Health Internet of Things (IoT) is paramount for engineers. This comprehension equips them to unleash the complete potential of IoT devices and transform raw data into actionable business insights. Our exploration extends from the fundamental principles of IoT analytics to delineating various types of analyses, and elucidates its multifaceted applications across diverse industries.
The concept of Healthcare 4.0, which is a recent emergence inspired by Industry 4.0, signifies a notable evolution in the healthcare sector. Healthcare is significantly more digitized than in previous decades. This transformation is evident in various advancements, such as the proliferation of technologies ranging from traditional X-rays and magnetic resonance imaging to more sophisticated techniques like computed tomography and ultrasound scans and the widespread adoption of electronic medical records.
For healthcare providers, understanding IoT analytics is no longer optional—it’s essential. By leveraging the power of IoT data, providers can move beyond traditional, reactive care models and unlock the full potential of preventive, data-driven healthcare. Whether it’s predictive care, real-time patient monitoring, or optimizing operations, IoT analytics holds the key to a healthier future.
Learn more
Amaral, L. A., de Matos, E., Tiburski, R. T., Hessel, F., Lunardi, W. T., & Marczak, S. (2016). Middleware technology for IoT systems: Challenges and perspectives toward 5G. In Modeling and Optimization in Science and Technologies (Vol. 8, pp. 333–367). Springer Verlag. https://doi.org/10.1007/978-3-319-30913-2_15
National University of Sciences and Technology, Islamabad, Pakistan, Joyia, G. J., Liaqat, R. M., Farooq, A., & Rehman, S. (2017). Internet of Medical Things (IOMT): Applications, Benefits and Future Challenges in Healthcare Domain. Journal of Communications. https://doi.org/10.12720/jcm.12.4.240-247
Jayaraman, P. P., Forkan, A. R. M., Morshed, A., Haghighi, P. D., & Kang, Y.-B. (2020). Healthcare 4.0: A review of frontiers in digital health. WIREs Data Mining and Knowledge Discovery, 10(2), e1350. https://doi.org/10.1002/widm.1350